فهرست مطالب
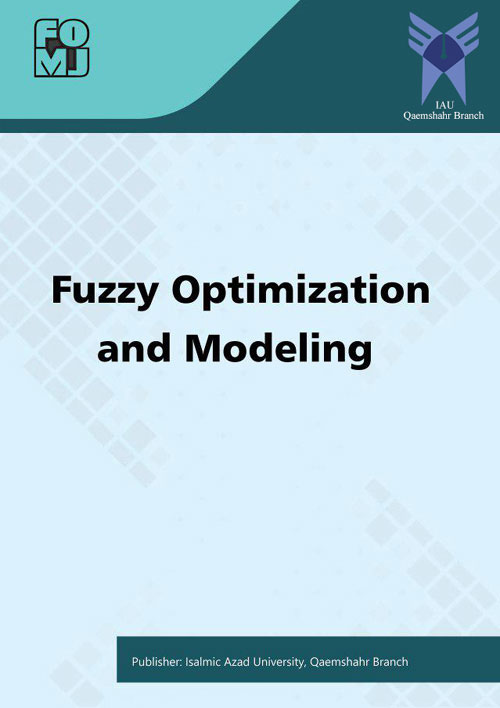
Fuzzy Optimzation and Modeling
Volume:2 Issue: 4, Autumn 2021
- تاریخ انتشار: 1400/10/28
- تعداد عناوین: 6
-
Pages 1-9In this paper, we improved the split step $ vartheta $ method to solve the stochastic differential equations. The strong convergence of this approximation for stochastic differential equations, whose drift and diffusion coefficients are globally Lipschitz continuous, are investigated. Furthermore, we analyze the stability in the mean square sense of our scheme by scalar stochastic differential equation with multi dimensional Wiener processes. The study of stability shows the mean square stability of the method for $ vartheta in [1/2, 1] $. Finally, we present some numerical examples to describe the methodology and implementation of the split step $ vartheta $ method to solve linear and nonlinear one dimensional stochastic differential equations and the Lotka-Volterra stochastic system.Keywords: Stochastic differential equations, Split step $vartheta$ method, Strong convergence, Mean square stability
-
Pages 10-19The integration of data envelopment analysis (DEA) approach and Malmquist productivity index (MPI) is one of the popular and powerful techniques in order to calculate of changes in productivity of homogeneous decision making units (DMUs) over different time periods. In this paper, an extended Malmquist productivity index will be presented that is capable to be employed in the presence of fuzzy data and linguistic variables. It should be noted that possibilistic programming (PP) as well as chance-constrained programming (CCP) approaches are applied to handle data ambiguity. The implementation of the proposed fuzzy Malmquist productivity index (FMPI) is illustrated by a numerical example under triangular fuzzy data. Finally, the results show the applicability and efficacy of the extended MPI to calculate the changes of productivity of DMUs under fuzzy environment.Keywords: Malmquist Productivity Index, Data envelopment analysis, Possibilistic Programming, fuzzy data
-
Pages 20-37
The stochastic data envelopment analysis (SDEA) is based on treating input values and output values as random variables. Typically, in research related to stochastic data envelopment analysis, variables are assumed to be independent of one another. It is likely that there will be a dependency structure between variables. We investigated the dependence structure between input variables and between output variables. To estimate this dependence structure, we used the Copula approach. Therefore, we have proposed a stochastic DEA model with a dependence structure called Copula-SDEA in this study and evaluated this model using both input-oriented and output-oriented models. We evaluated the proposed models using real data from 10 car companies. The implementation of the proposed model showed that, different results can be drawn when taking into account the dependence structure between stochastic inputs and (or) outputs. Additionally, a comparison of Copula-SDEA models with the SDEA model revealed that the evaluation of the efficiency of DMUs with Copula-SDEA models differed significantly from the SDEA model. Moreover, the results indicate that in both input- and output-oriented models, considering the dependence structure between inputs is more important than considering the dependence structure between outputs.
Keywords: Stochastic Data envelopment analysis, Copula Theory, Copula-SCCR -
Pages 38-51The Analytic Hierarchy Process (AHP) is one of the most widely used techniques to determine the priority weights of alternatives from pairwise comparison matrices. Several fuzzy and intuitionistic fuzzy extensions of AHP have been proposed in the literature. However, these extensions are not appropriate to present some real-life situations. For this reason, several researchers extend the AHP to the Pythagorean Fuzzy Analytic Hierarchy Process (PFAHP). In the existing methods, an interval-valued Pythagorean fuzzy pairwise comparison matrix is transformed into a crisp matrix. Then crisp AHP is applied to obtain the normalized priority weights from the transformed crisp matrix. However, it is observed that the transformed crisp matrix, obtained on applying the step of existing methods, violates the reciprocal propriety of pairwise comparison matrices, and the obtained normalized priority weights are the weights of non-pairwise comparison matrices. Therefore, this paper discusses the shortcomings of the existing method, and a modified method is proposed to overcome these shortcomings. Finally, based on a real-life decision-making problem, the superiority of the proposed method over the existing method is shown.Keywords: Pairwise comparison matrix, AHP, Pythagorean Fuzzy Numbers
-
Pages 52-64
Data Envelopment Analysis (DEA) is an impeccable approach based on mathematical programming for the efficiency measurement of homogeneous Decision-Making Units (DMUs). One of the topics of interest in data envelopment analysis (DEA) is the sensitivity and stability analysis of a specific DMU that determines ranges within which all data may be altered for any DMU before a reclassification from efficient to inefficient status (or vice versa) happens. In many real-world applications, the managers to estimate the under supervision DMUs encounter stochastic data and require a way to deal with the sensitivity analysis of DMUs with this special data. In DEA, efficient DMUs are of primary importance as they define the efficient frontier. The intent of this paper is to present the sensitivity analysis with stochastic data for efficient DMUs when inputs and outputs are stochastic and variations in the data are simultaneously considered for all DMUs. The models explained in this paper for treating sensitivity analysis in DEA are expanded by according them chance-constrained programming formulations. The ordinary route used in chance-constrained programming is followed here by replacing these stochastic models with their deterministic equivalents. The optimal solution of these models leads to allowable input/ output variations.
Keywords: Data envelopment analysis, Efficiency sensitivity analysis, stability region, Stochastic data -
Pages 65-74Data envelopment analysis (DEA) is a non-parametric technique to measure and evaluating the relative efficiencies of the set of homogenous decision making units (DMUs) with multiple inputs and multiple outputs. Traditional DEA models assume that inputs and outputs to be continuous, real-valued data. In many occasions, inputs or outputs can only take integer values. Therefore, DEA models can not be used for determining efficiency score of DMUs. The current paper applies of the modified classic DEA models to obtain attractiveness and progress in integer-valued technologies. For this aim, in the first phase, the efficiency score of all DMUs are measured and the efficient and inefficient units are determined. Then, in the second phase, we remove the main efficient frontier that corresponds to the efficient units, and then we create a new efficient frontier as the second layer efficient frontier of the remaining units (units inefficient). With repeat this process, we find the next layers until there is no any unit left. Finally, the attractiveness and progress of each unit is calculated from one efficient level relative to the other efficient level.Keywords: Data envelopment analysis, Mixed Integer Linear Programming( MILP), Attractiveness, progress